
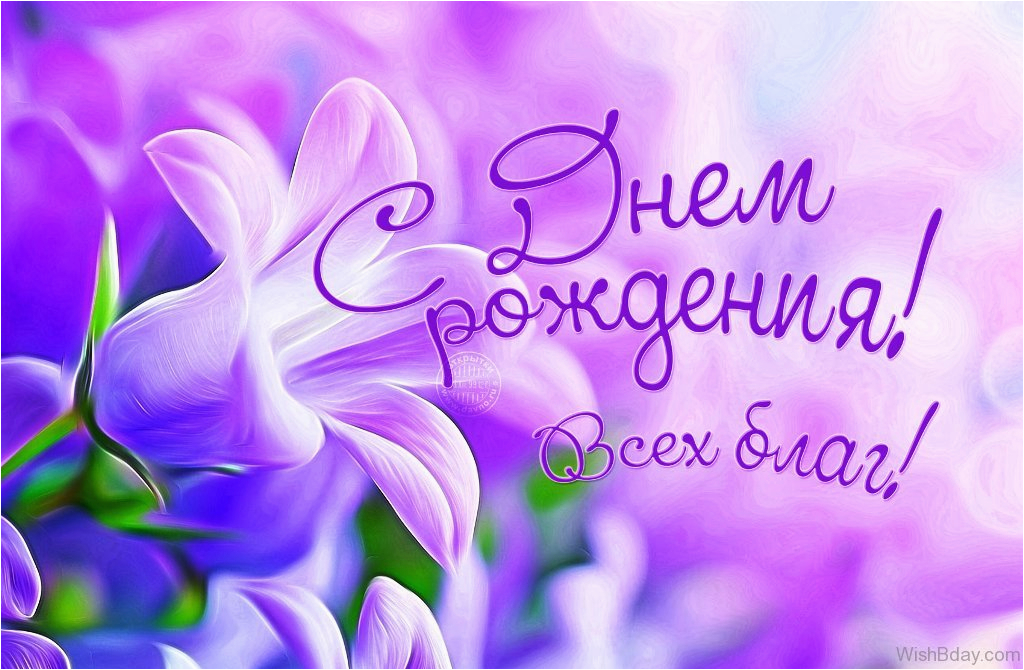
It takes a target-language text which is gender-biased in one way and biases it the other way (for example from male doctor to female doctor). Rewriting is an additional step which happens after the translation is produced but before it is shown to a human user. Instead, they allow their machine-learned models to produce gender-biased translations as before, and they rewrite them now. They’re no longer pre-editing the training data. Then, sometime around the year 2020, they changed their mind and adopted a different strategy. The translator is then able to take an input, insert the same invisible gender tokens into it, and produce separate “masculine” and “feminine” translations.
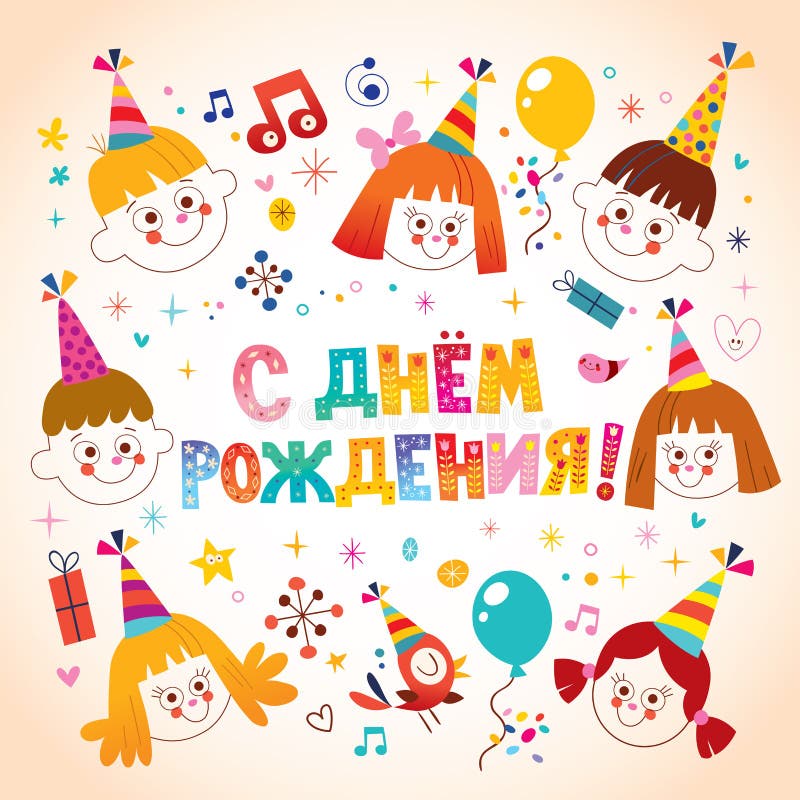
Their original solution from 2018 was based on the idea that you “pre-edit” your training data by inserting invisible gender tokens into the source-language side, and then you machine-learn your translator from that. They have obviously experimented with different strategies and even changed course completely at least once. How does Google’s gender-specific translation feature work inside? Fortunately, Google is not too secretive (unlike DeepL), they have published blog posts and academic papers which explain in broad terms how they have done it. So the interesting question is, how do the two compare? What’s same, what’s different, and which is better? In this article I’m going to discuss how Google is “doing” gender-specific translations and talk about the differences between their approach and Fairslator’s. Gender-specific translation in Google Translate.Īs you may know, Fairslator has a feature like this too, although in different language pairs (currently: English to French, German and Czech).
